Person ID Dataset
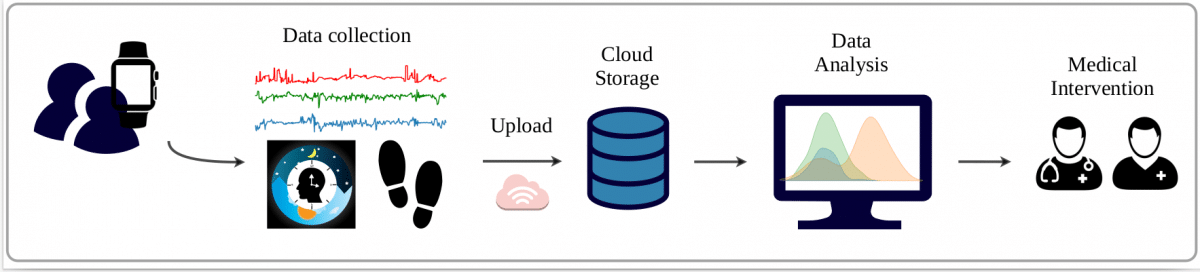
The Person ID dataset includes data from a Samung Gear S3 Frontier Smart Watch for 20 volunteers, recorded over a period of 20 or more days, during the ePrevention Project (https://eprevention.gr/). The continuously recorded data included 1) angular velocity (gyroscope sensor), 2) linear acceleration (accelerometer), 3) heart rate (PPG sensor), and 4) walking and sleeping information.
Abstract: In this work, we explore the discriminating ability of short-term signal patterns (e.g. few minutes long) with respect to the person identification task. We focus on signals recorded by simple wearable devices, such as smart watches, which can measure movements (accelerometer and gyroscope sensors) and biosignals (heart rate monitor). To address the person identification problem, we develop a deep neural network, based on one-dimensional convolutions, which receives raw signals from three different smartwatch sensors and predicts the person wearing the smartwatch. Experimental results indicate that even with signals from wearable sensors collected at intervals of only 10 minutes, different users can be identified with notably high accuracy, revealing the existence of distinct short-term patterns of movement and heart rate between different persons.
The total size of the dataset is 20GB. Click here to download the dataset.
Reference to:
Communication:
For more information or details regarding the dataset contact:
George Retsinas, PhD
IRAL, National Technical University of Athens
Email: gretsinas@central.ntua.gr